TRUST OKOROEGO
Trust Okoroego, Reporting Senior Application Expert at Holcim EMEA Digital Center shares with us how Data Lake technology is helping us accelerate the digital transformation
The benefits of data lake strategy
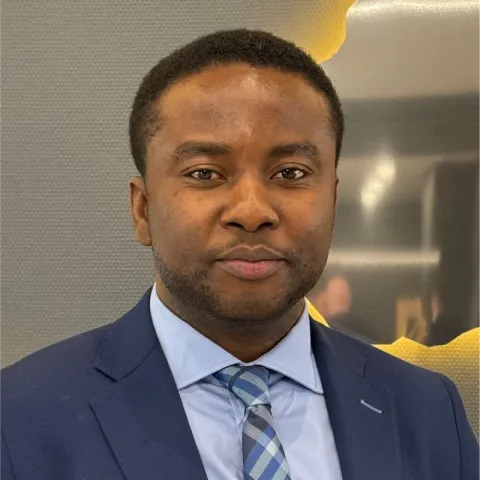
What is Data Lake?
Data Lake is a centralized repository that allows the storing of structured and unstructured data at any scale and velocity at a very low cost. Data can be stored as-is from source systems, without having to first structure the data.
Unlike a data warehouse, the structure of the data or schema is not defined at extraction time. This makes it possible to quickly collect and store all types of data without spending time on careful schema design or the need to know what questions need to be answered in the future.
Why a Data Lake strategy?
We are currently experiencing massive data growth resulting from the organization's digital initiatives. Managing this huge amount of data growth and supporting a wide range of analytical use cases requires a complementary solution that can scale to any data size and at a very low cost.
A second reason is the need for a centralized source of truth that facilitates easy access to quality data. A common challenge we have seen from our customers is that it is sometimes difficult to find the right data they need, how to access it and understanding the business meaning.
A third reason is the need for real time data to support real time business decisions without compromising the performance of the source transactional systems.
Benefits of a Data Lake strategy
- Data Democratization: Anyone in the organization can easily search for quality certified data, understand their lineage (sources), the business meaning and easily request access to the data. This will lead to faster, better decision making across the organization.
- Advanced Analytics: It opens the possibilities for a wide range of advanced analytics use cases like Machine learning model training at scale, forecasting, user facing analytics, real time analytics, etc.
- Unlimited Scalability: There is practically no limit to the amount of data that can be stored, thus we can store as much historical data as possible. It also allows us to scale processing of these massive data horizontally at a very low cost.
Key factors for a successful Data Lake implementation
One of the key success factors is having a clear a requirement. A clear requirement will guide the overall Data Lake architectural design and implementation, thus increasing the success rate. These requirements could be broken intro three:
- Business requirements: Who are the primary end users for the data lake? What do they need to achieve via the data lake and what additional potential benefit can they derive from the data lake?
- Data Requirements: What sources will feed the data lake? What type of data integration will be required?
- Analytical requirements: How will the data be accessed and analyzed?
Another key factor is defining Data Governance from the beginning of the implementation. This involves deciding how permissions will be managed, how the data will be cataloged, identifying which falls under Personally Identifiable information(PII), how to manage GDPR requirements and how to measure and ensure data quality. Without a proper data governance in place, a data lake will easily turn into an unusable data swamp.
And lastly, ensuring the stability and availability of the data platform through DataOps. This involves automating the platform infrastructure deployment and management, setting up automated monitoring and alerting rules for both the infrastructure and the data pipeline workflows.